Abstract
Introduction and hypothesis
Obstetric anal sphincter injury (OASI) is a complication with substantial maternal morbidity. The aim of this study was to develop a machine learning model that would allow a personalized prediction algorithm for OASI, based on maternal and fetal variables collected at admission to labor.
Materials and methods
We performed a retrospective cohort study at a tertiary university hospital. Included were term deliveries (live, singleton, vertex). A comparison was made between women diagnosed with OASI and those without such injury. For formation of a machine learning-based model, a gradient boosting machine learning algorithm was implemented. Evaluation of the performance model was achieved using the area under the receiver-operating characteristic curve (AUC).
Results
Our cohort comprised 98,463 deliveries, of which 323 (0.3%) were diagnosed with OASI. Applying a machine learning model to data recorded during admission to labor allowed for individualized risk assessment with an AUC of 0.756 (95% CI 0.732–0.780). According to this model, a lower number of previous births, fewer pregnancies, decreased maternal weight and advanced gestational week elevated the risk for OASI. With regard to parity, women with one previous delivery had approximately 1/3 of the risk for OASI compared to nulliparous women (OR = 0.3 (0.23–0.39), p < 0.001), and women with two previous deliveries had 1/3 of the risk compared to women with one previous delivery (OR = 0.35 (0.21–0.60), p < 0.001).
Conclusion
Our machine learning-based model stratified births to high or low risk for OASI, making it an applicable tool for personalized decision-making upon admission to labor.



Similar content being viewed by others
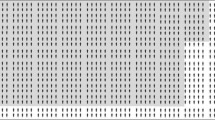
References
Stock L, Basham E, Gossett DR, Lewicky-Gaupp C. Factors associated with wound complications in women with obstetric anal sphincter injuries (OASIS). Am J Obstet Gynecol. 2013;208:327.e1–6.
Fernando RJ, Sultan AH, Kettle C, Thakar R. Methods of repair for obstetric anal sphincter injury. Cochrane Database Syst Rev. 2013;12:CD002866.
Handa VL, Blomquist JL, McDermott KC, Friedman S, Munoz A. Pelvic floor disorders after childbirth: effect of episiotomy, perineal laceration and operative birth. Obstet Gynecol. 2012;119:233–9.
Fitzpatrick M, O’Herlihy C. Short-term and long-term effects of obstetric anal sphincter injury and their management. Curr Opin Obstet Gynecol. 2005;17:605–10.
Richter HE, Nager CW, Burgio KL, Whitworth R, Weidner AC, Schaffer J, et al. Incidence and predictors of anal incontinence after obstetric anal sphincter injury in primiparous women. Female Pelvic Med Reconstr Surg. 2015;21:182–9.
Nordenstam J, Altman D, Brismaz S, Zetterstrצּm J. Natural progression of anal incontinence after childbirth. Int Urogynecol J Pelvic Floor Dysfunct. 2009;20(9):1029–35.
Wegnelius G. Hammarstrצm M. complete rupture of anal sphincter in primiparas: long term effects and subsequent delivery. Acta Obstet Gynecol Scand. 2011;90(3):258–63.
Ott J, Gritsch E, Pils S, et al. A retrospective study on perineal lacerations in vaginal delivery and the individual performance of experienced midwives. BMC Pregnancy Childbirth. 2015;15:270.
Low LK, Zielinski R, Tao Y, Galecki A, Brandon CJ, Miller JM. Predicting birth-related levator ani tear severity in primiparous women: evaluating maternal recovery from labor and delivery (EMRLD study). Open J Obstet Gynecol. 2014;4:266–78.
Andrews V, Sultan AH, Thakar R, Jones PW. Risk factors for obstetric anal sphincter injury: a prospective study. Birth. 2006;33:117–22.
Baghestan E, Irgens LM, Bordahl PE, Rasmussen S. Trends in risk factors for obstetric anal sphincter injuries in Norway. Obstet Gynecol. 2010;116:25–34.
Deo RC. Machine learning in medicine. Circulation. 2015;132(20):1920–30.
Obermeyer Z, Emanuel EJ. Predicting the future - big data, machine learning and 509 clinical medicine. N Engl J Med. 2016;375(13):1216–9.
Guedalia J, Lipschuetz M, Novoselsky Persky M, et al. Real-time data analysis using a machine learning model significantly improves prediction of successful vaginal deliveries. Am J Obstet Gynecol. 2020;223(3):437.
McPherson KC, Beggs AD, Sultan AH, Thaker R. Can the risk of obstetric anal sphincter injuries (OASIs) be predicted using a risk-scoring system? BMC Research Notes. 2014;7:471.
Meister MR, Cahill AG, Conner SN, Woolfolk CL, Lowder JL. Predicting obstetric anal sphincter injuries in a modern obstetric population. Am J Obstet Gynecol. 2016;215(3):310.e1–7.
Committee on Practice Bulletins-Obstetrics. ACOG practice bulletin no. 198: prevention and Management of Obstetric Lacerations at vaginal delivery. Obstet Gynecol. 2018 Sep;132(3):e87–e102.
Youden WJ. Index for rating diagnostic tests. Cancer. 1950;3(1):32–3.
Prokhorenkova L, Gusev G, Vorobev A, Dorogush AV, Gulin A. Catboost: unbiased boosting with categorical features. In: Proceedings of the 32nd International Conference on Neural Information Processing Systems, Montréal, QC, Canada, pp. 6639–6649
Lundberg SM, Erion G, Chen H, et al. From local explanations to global understanding with explainable AI for trees. Nature Machine Intell. 2020;2(1):56–67.
Valsky DV, Cohen SM, Lipschuetz M, et al. Third- or fourth-degree intrapartum anal sphincter tears are associated with Levator Ani avulsion in Primiparas. J Ultrasound Med. 2016;35(4):709–15.
Ying T, Li Q, Xu L, Liu F. Hu B. three-dimensional ultrasound appearance of pelvic floor in nulliparous women and pelvic organ prolapse women. Int J Med Sci. 2012;9(10):894–900.
Dietz HP, Abbu A, Shek KL. The levator-urethra gap measurement: a more objective means of determining levator avulsion? Ultrasound Obstet Gynecol. 2008;32:941–5.
Dietz HP, Bhalla R, Chantarasorn V, Shek KL. Avulsion of the puborectalis muscle is associated with asymmetry of the levator hiatus. Ultrasound Obstet Gynecol. 2011;37:723–6.
Hickman LC, Propst K. Accurate diagnosis and repair of obstetric anal sphincter injuries: why and how. Am J Obstet Gynecol. 2020;222(6):580.e1–580.e5.
Financial support
None
Author information
Authors and Affiliations
Contributions
Chill: Project development, data collection, data analysis, manuscript writing and editing.
Guedalia: Project development, data collection, data analysis, manuscript writing and editing.
Lipschuetz: Data collection, data analysis, manuscript editing.
Shimonovitz: Data analysis, manuscript editing.
Unger: Data analysis, manuscript editing.
Shveiky: Manuscript editing, data analysis.
Karavani: Project development, data collection, data analysis, manuscript writing and editing.
Corresponding author
Ethics declarations
Conflict of interest
The authors have no conflict of interest to report.
Additional information
Publisher’s note
Springer Nature remains neutral with regard to jurisdictional claims in published maps and institutional affiliations.
Rights and permissions
About this article
Cite this article
Chill, H.H., Guedalia, J., Lipschuetz, M. et al. Prediction model for obstetric anal sphincter injury using machine learning. Int Urogynecol J 32, 2393–2399 (2021). https://doi.org/10.1007/s00192-021-04752-8
Received:
Accepted:
Published:
Issue Date:
DOI: https://doi.org/10.1007/s00192-021-04752-8